Human language is inherently complex. It follows intricate rules, is full of exceptions, and is shaped by subtle nuances and cultural context. For a long time, it seemed unimaginable that machines could understand and process language meaningfully. But with advances in natural language processing—especially in AI translation—this belief is being challenged.
AI translation uses machine learning and neural networks to automatically convert text from one language to another. Unlike older machine translation systems that relied on rule-based or statistical approaches, today’s AI-driven solutions can learn from large datasets and improve over time. This not only increases translation speed but also boosts accuracy, scalability, and affordability.
What Does AI Translation Mean?
AI translation refers to the use of artificial intelligence—particularly deep learning and neural networks—to perform translations. The systems are designed to improve continuously by analyzing large sets of multilingual content and adapting based on human feedback.
In practice, this means:
- Higher-quality results over time
- More contextual translations
- Flexibility across use cases, from casual chats to technical documentation
These systems are now part of a more advanced evolution of traditional machine translation. Tools like DeepL, Google Translate, and services integrated with Locize offer solutions that blend automation with real-world usability.
How AI Translation Works: From Rules to Neural Networks
The history of AI translation is characterized by several technological development steps. In the 1950s, the first egel-based systems were created, which were based on manually defined grammar structures and bilingual dictionaries. However, their performance remained limited: Language is difficult to force into fixed rules, as it relies heavily on context and shades of meaning.
In the 1990s, statistical machine translation (SMT) ushered in a new era. It used large quantities of bilingual texts to calculate probabilities for word and phrase combinations.
This made it possible to generate translations based on frequently occurring patterns. A significant advance over rigid sets of rules. Nevertheless, SMT remained prone to errors, especially when dealing with idiomatic expressions, rare terms or complex contexts.
The transition to neural machine translation (NMT) from around 2015 marked a real turning point. Neural networks make it possible to analyze not just individual words, but entire sentences or paragraphs in context. This makes it possible to produce translations that appear much more natural and coherent. The systems take the context into account and are guided by patterns that they have learned from extensive training data - similar to a person who derives meaning from experience and environment.
The key advantages of modern NMT systems include:
- Context-related translation of entire sentences instead of isolated words
- Coherence in the text flow, even across sentence boundaries
- Adaptability through fine-tuning, e.g. for specialist areas such as medicine, law or technology
- Learning ability through real-time feedback, for example in systems such as Modern MT
AI translation systems evolved through several phases:
- Rule-based MT (1950s–1990s): Manual grammar rules and dictionaries
- Statistical MT (1990s–2015): Probabilistic models learned from bilingual corpora
- Neural MT (2015–today): Deep learning with full sentence/context understanding
In particular, the so-called Transformer model, introduced by Google in 2017, has significantly improved the quality of machine translations. It enables particularly effective context processing and has established itself as the basis for many of today's translation systems.
Modern AI translation is also based on artificial neural networks. The systems first convert spoken or written language into sequences of numbers. This is a prerequisite for computers to be able to process language. The translation process follows the principle of an encoder-decoder architecture.
First, the encoder takes on the task of converting a sentence in the source language (e.g. German) into a mathematical representation.
This is then interpreted by the decoder and transferred into the target language (e.g. Spanish).
A simple example to illustrate this:
The sentence “I have a cat” is converted by the system into the number sequence 251, 2134, 953, 4.
From this, the model calculates a new sequence of numbers - such as 4356,7654, 76, 5325 - which is then output by the decoder as “tengo un gato”.
- Input: “Ich habe eine Katze” → Encoded as numeric vector
- Output: “tengo un gato” → Decoder reconstructs output from encoded meaning
Despite all the progress, one limitation remains: Even the most advanced AI does not “understand” language in the human sense.
It works purely on the basis of probabilities. The statistically most probable result is output. As a result, words may be omitted or new content may be “hallucinated” that is not even present in the original text.
These technical limitations are relevant when it comes to evaluating translation quality in direct comparison with human specialist translators.
AI doesn’t understand language. It generates likely translations based on statistical probability, which may result in omissions, hallucinations, or misinterpretations.
Why AI Translation Is a Game-Changer
The use of AI translation (i.e. AI-supported translation) has a number of clear advantages over traditional, particularly purely human translation methods. These include significantly higher speed, lower costs, the ability to scale large volumes of text and consistent quality - especially for standardized or recurring content.
More about it in this blog what is translation?
High speed and scalability
AI systems process large volumes of text in a very short time, far faster than human translators would be able to.
Within seconds, thousands of product descriptions, website content or other text forms can be translated simultaneously into several languages.
This reaction speed is invaluable, especially for time-critical applications such as live communication via chat or email. What's more, AI translation is available around the clock and is not subject to fatigue.
Cost efficiency with high text volumes
Once an AI translation system has been set up or licensed, additional translations can be implemented with minimal additional effort.
In contrast to human translation, there are no ongoing personnel costs. Practical experience and studies show that the costs per word can be reduced by up to 50 to 70 percent, depending on the application, and AI translation creates economic leeway that would be almost impossible to achieve with conventional means, especially for large volumes of text or limited budgets.
Uniformity and terminological consistency
A decisive advantage lies in the consistent use of terminology. Once the AI translation has learned how to translate a specific term, it applies this translation consistently and without stylistic deviations or careless mistakes.
The high degree of reliability is particularly important in sensitive areas such as technical documentation, which requires precise configuration, for example through company-specific glossaries or targeted training within the translation software environment.
If the system is set up accordingly, it guarantees consistent language use throughout. Regardless of daily form or stress, which can play a role with human translators.
Permanent availability and robust performance
AI-Translation is ready for use at any time. They require no pauses, make no typing errors and deliver results on demand, whether for individual sentences or millions of text modules. Even with massive amounts of data, the processing time remains stable and grows only minimally with the volume, making it possible to integrate translations seamlessly into workflows.
Examples range from the automated translation of incoming customer queries in customer service to the ongoing localization of social media content.The use of modern software localization tools also makes it possible to efficiently adapt complex applications for international markets - including user interfaces, help content and system messages.
The use of modern software localization tools also makes it possible to efficiently adapt complex applications for international markets - including user interfaces, help content and system messages.
With Locize, you can integrate automatic translation directly into your CI/CD process. New keys (via i18next saveMissing, CLI, or API) are translated instantly and marked as fuzzy for human review. You can learn more about it here.
Where AI Translation Is Used
- Customer support: Automated replies and knowledge base localization
- E-commerce: Product listings and reviews translated instantly
- Internal docs: Translating technical manuals or SOPs across offices
- Software & apps: Real-time multilingual UI/UX updates using tools like Locize
Example: At Networkme, developers use Locize to manage six web applications. Marketing teams translate copy without waiting on code releases—thanks to AI-assisted workflows and real-time editing.
AI translation is already transforming day-to-day operations across industries—especially where multilingual communication is essential.
Customer Service and Support
AI translation helps companies overcome language barriers in real time. Support queries via email or chat can be automatically translated for the support team and back to the user. With Locize, FAQs and helpdesk articles can be localized and updated continuously without waiting on developers.
Example: Companies like Withlocals and Wuxus use Locize to translate support platforms and customer-facing portals with ease, enhancing user experience across languages.
E-commerce and Website Localization
Product descriptions, customer reviews, and even UI strings can be instantly translated using AI. For time-sensitive content updates, AI delivers speed while human reviewers ensure tone and branding stay intact.
Example: carVertical uses Locize to manage vehicle report content in over 30 languages, enabling rapid market expansion with consistent messaging.
Internal Communication and Documentation
Multinational teams benefit from AI-powered translation of project updates, onboarding materials, and policy documents. Platforms like Microsoft Teams already integrate this, but you can go further by using Locize to manage documentation in a centralized, reviewable way.
Example: ke-chain.com and Logiscool translate technical documentation and learning content using Locize’s developer-friendly APIs.
Legal, Medical, and Technical Drafts
For sensitive or complex material, AI provides a quick first draft—perfect for time-saving. Post-editing by professionals ensures the final output meets quality and regulatory requirements.
Example: Geoimpact and Shift Cryptosecurity use Locize to manage regulated and sensitive translations in a streamlined workflow.
Everyday Use Cases
Social media teams use AI to monitor global brand mentions. Tools like Locize allow fast translation of posts and replies. Video conferencing tools integrate AI to provide live captions and multilingual subtitles.
Example: Tontine Trust and Nazata Bio rely on Locize to update public-facing platforms quickly, across multiple languages and content types.
The rule: the simpler the structure, the more reliable the AI. For nuanced or high-stakes content, human review remains essential.
Real-World Workflow: AI Translation with Locize
A typical developer-friendly setup might look like this:
- Define translation keys in your codebase (e.g., with i18next)
- Push them to Locize using saveMissing, CLI, or API
- Enable automatic AI translation in Locize (e.g., using OpenAI, DeepL, Gemini)
- Keys are marked as fuzzy and filtered for review
- Reviewers validate or correct translations in the Locize editor
- Fetch translations via CDN/API or bundle into your app
This AI-assisted, human-perfected approach delivers speed without sacrificing quality.
Bonus: You can use tags likeai-generated
or enable Locize’s Review Workflow for formal approval stages.
Man vs. machine: who translates more reliably?
Despite considerable progress, AI translation is not yet on a par with the work of professional translators. In many cases, the systems achieve a high level of formal quality and impress with their grammar and fluency. Particularly in the case of factual, clearly structured texts, AI now delivers results that at first glance can hardly be distinguished from human translations, but content-related weaknesses become apparent where context, cultural nuances or ambiguities play a role.
Although AI systems analyze the context of individual words, they do not always interpret it correctly. For example, the German word “Band” - depending on the context, music group, book series or strip of fabric - can be interpreted incorrectly.
A practical example: the phrase “drive on the right” was automatically interpreted as a political statement, which completely distorted the meaning.
Stylistic subtleties are another challenge. AI translation does not recognize target groups or tonality and often translates texts in a factually neutral style. Regardless of whether it is marketing content, internal communication or emotional messages. Humor, irony or wordplay are regularly lost or rendered inappropriately.
AI translation also reaches its limits in the creative field. Emotions, vivid language or persuasive effects are only partially successful. An automatically generated advertising slogan often remains pale, while a human copywriter controls the linguistic effect in a targeted manner.
The situation is similar with specialist legal or medical texts: although AI systems use terminology consistently, they lack a deeper understanding of subtleties that may be essential in the respective specialist context.
It is particularly problematic with less widely used languages. Here, there is often a lack of sufficient training material, which has a noticeable impact on quality. While AI translation delivers good results for common language pairs, the gap remains noticeable for minority languages.
All of this shows that AI is ideal for quick, initial drafts. However, it can only achieve the final level of quality in combination with human expertise. In practice, a hybrid model is therefore gaining acceptance: AI translation takes care of the initial translation, while humans fine-tune the language and content. This produces high-quality translations faster and more cost-effectively than purely manual work - while maintaining the same level of quality.

Current tools for AI translation
Specialized AI translation systems such as Google Translate and DeepL are among the best-known tools on the market when it comes to AI translation. Google Translate has been around since 2006, while DeepL was developed from the Linguee database in 2017.
In July 2024, DeepL was further optimized to make translations sound more natural.
An indication that, despite progress, machine translations are not yet at the level of human language.
Generative AI models such as ChatGPT, Gemini or Perplexity are suitable for creative processes, research or idea generation, but they are not specialized AI translation tools.
Their results lag far behind those of professional translation tools. Especially for specialist texts, less common languages or stylistically demanding content.
The market is dominated by large tech providers as well as specialized platforms and open source initiatives. Here is a compact overview of the key AI translation tools currently available:
- DeepL – High-quality translations for European languages, excellent style
- Google Translate – Wide language coverage, strong real-time support
- Microsoft Translator – Office/Teams integration, Azure ecosystem
- Amazon Translate – Built for scalable, secure enterprise use
- Meta’s NLLB-200 – Covers 200 languages, supports low-resource regions
- Open-source – Projects like Opus-MT, Marian NMT for on-premise deployments
What about AI translation and the law?
There is no question that the use of AI translation raises legal and ethical issues that companies cannot ignore, again in all areas where AI is gaining in importance. Data protection is a particular focus. If personal or confidential information is processed via free online translators such as Google Translate or DeepL Free, there is a risk that this data will be used to train the AI or stored on servers.
This could potentially make sensitive content public. For business applications, providers such as DeepL or Microsoft therefore also offer Pro versions with contractually guaranteed data protection.
Companies should always check whether an order processing contract is necessary and, if necessary, anonymize the content.
Security aspects such as potential hacker attacks or data leaks must also be considered, and copyright is another challenge in AI translation.
Translations are only considered protectable works if they are the result of a human, creative effort. AI-generated translations usually do not meet this requirement.
In addition, the legal situation surrounding the training of AI models with copyright-protected content remains unclear. Companies should therefore always respect the rights to the original text when publishing machine-translated texts. In terms of liability, the question arises as to who is responsible for damages resulting from incorrect translations by AI translation.
It is currently assumed that responsibility lies with the user.
Companies that use AI must check the results before they are published, so machine translation should not be used as the final version for sensitive content such as contracts, operating instructions or legal texts. Post-editing by qualified specialist translators is recommended in order to minimize risks.
Ethical aspects must not be ignored either. AI models reproduce potential distortions from their training data.
For example, cases have been reported in which gender-neutral terms were translated stereotypically. This can result in unintentional discrimination. There is also the question of how the use of AI translation will change the profession of translator in the long term.
Although technology is increasingly taking over routine tasks, complex, creative or culturally sensitive translations will remain in human hands. It is important to shape the change fairly - for example through further training and transparent communication with end users.
Particularly in industries with high quality standards, it should be clearly recognizable whether a translation has been produced by a machine or by a human professional, and as long as legal standards, such as those provided for in the EU AI Act, are not yet in place, it is up to companies to ensure that AI translation is handled responsibly.
This includes binding guidelines, quality assurance processes and a critical look at the limits of machine systems.
Future Trends in AI Translation
AI translation is evolving rapidly. New models and use cases continue to shape the landscape.
Generative language models like GPT-4 are beginning to rival traditional NMT systems, providing surprisingly strong results due to their deep contextual understanding.
Translation is increasingly becoming a background feature in broader AI ecosystems—what some call "translation as a feature."
Real-time and multimodal translation is gaining traction. Tools like Skype Translator and Zoom's captioning system already support live, multilingual communication.
In the future, AI will translate spoken language, text, and images simultaneously—e.g., street signs in AR glasses or subtitles in video calls. Meta’s SeamlessM4T is a promising step in that direction.
Expanding language coverage is also a top priority. Projects like NLLB-200 and Google’s 1,000-language initiative aim to support minority and endangered languages.
Techniques like zero-shot translation help models handle new languages with minimal data.
AI systems will also improve at maintaining context across long documents and referencing external world knowledge, such as proper names.
Translation will run invisibly in the background of platforms like YouTube, Reddit, or content management systems, using APIs as simple to call as cloud storage.
As usage grows, quality standards become more important. ISO norms like DIN ISO 5060 are emerging to define benchmarks. Labels indicating whether a translation was AI-generated or human-reviewed will become common.
The most likely scenario? A hybrid future: AI will handle routine tasks, while humans ensure tone, nuance, and cultural fit. Creative content like ads and literature will remain human-led. The key to success lies in using AI not as a replacement—but as a powerful tool.
Generative models (LLMs): Translation becomes part of large language models with strong contextual understanding
Real-time and multimodality: Translations in conversations, text, images and speech simultaneously
Expanded language coverage: Focus on minority languages and dialects through new training methods
Context processing: Consistent translations across entire documents and thematic contexts
Invisible integration: Translation as a background service in platforms, apps and CMS systems
Quality standards and norms: Certifications and objective evaluation procedures for AI translations
Hybrid working model: Combination of AI efficiency and human control for the best possible quality
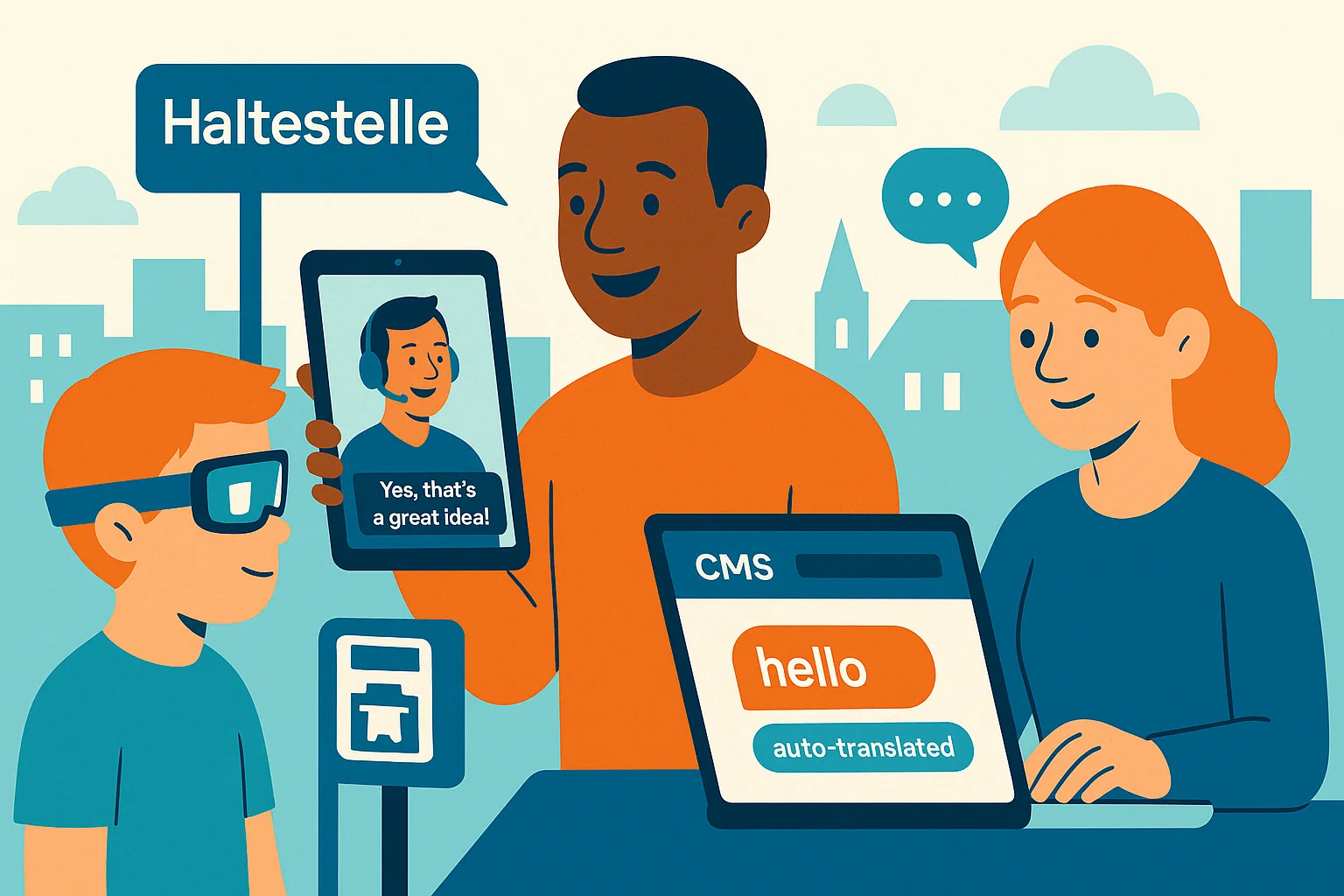